Artificial Intelligence and Data Science are two of the most prominent forces of the technological revolution. Their integration has profound implications for how organizations conduct their operations and make choices. Artificial Intelligence is a technique that uses algorithms to simulate human intelligence. Data Science deals with the management of vast datasets to ascertain insightful patterns. Jointly, they advance and strengthen data analytic features, foster innovation, and present deep opportunities in different fields. To understand the possibilities of these two interconnected fields, this article explores the interaction between Artificial Intelligence and Data Science.
Understanding Artificial Intelligence and Data Science
Artificial Intelligence or AI can be defined as the ability of machines to execute functions that are associated with human intelligence. AI is a broad concept that encompasses various technologies, including machine learning, where systems improve over time through experience. It also includes natural language processing, which teaches machines to understand and interpret human language. AI has found use in image and speech recognition, self-driving cars, and recommendation systems.
Data Science will also embrace the discovery of information and knowledge from structured and unstructured data. It uses mathematics, computers, and statistics to analyze big and small data to provide conclusions and recommendations for business solutions. Data Science is a set of procedures that involves data gathering, pre-processing, processing, as well as data presentation. The main purpose is to reveal the patterns and trends essential for business analytics and the formation of strategies for the company.
The Synergy Between Artificial Intelligence and Data Science
Enhanced Data Analysis
Data Science receives an appreciable increase in capacity to process data through the application of Artificial Intelligence. The use of concepts such as machine learning models allows AI to analyze large amounts of information at a vastly faster pace. This results in much higher degrees of precision compared to manually implemented methods. Such algorithms help detect patterns and relationships in data that a human might not interpret using his/her eyes. The high level of data analysis also means that organizations are able to gain better insights in relation to data analysis and decision-making.
Predictive Analytics
AI is strategic in predictive analytics mainly due to the ability to analyze historical data towards future Statistics. Artificial Intelligence can be mainly classified into two categories. Machine Learning finds patterns in historical data sets to make probable estimations for future occurrences. It also identifies opportunities or threats based on these patterns. This predictive capacity benefits various industries, such as finance and healthcare, by enabling better planning for market changes.
Automated Insights
Another great feature of AI in Data Science is the possibility of using it to produce insight automatically. AI-based systems can analyze data and prepare reports without human intervention. This capability saves a significant amount of time and effort. Leading to real-time decision-making, while saving data scientists’ time to work on more sophisticated projects. In this way, AI optimizes the processes of analysis and speeds up the receipt of results, freeing the analyst from repetitive tasks.
Challenges and Considerations
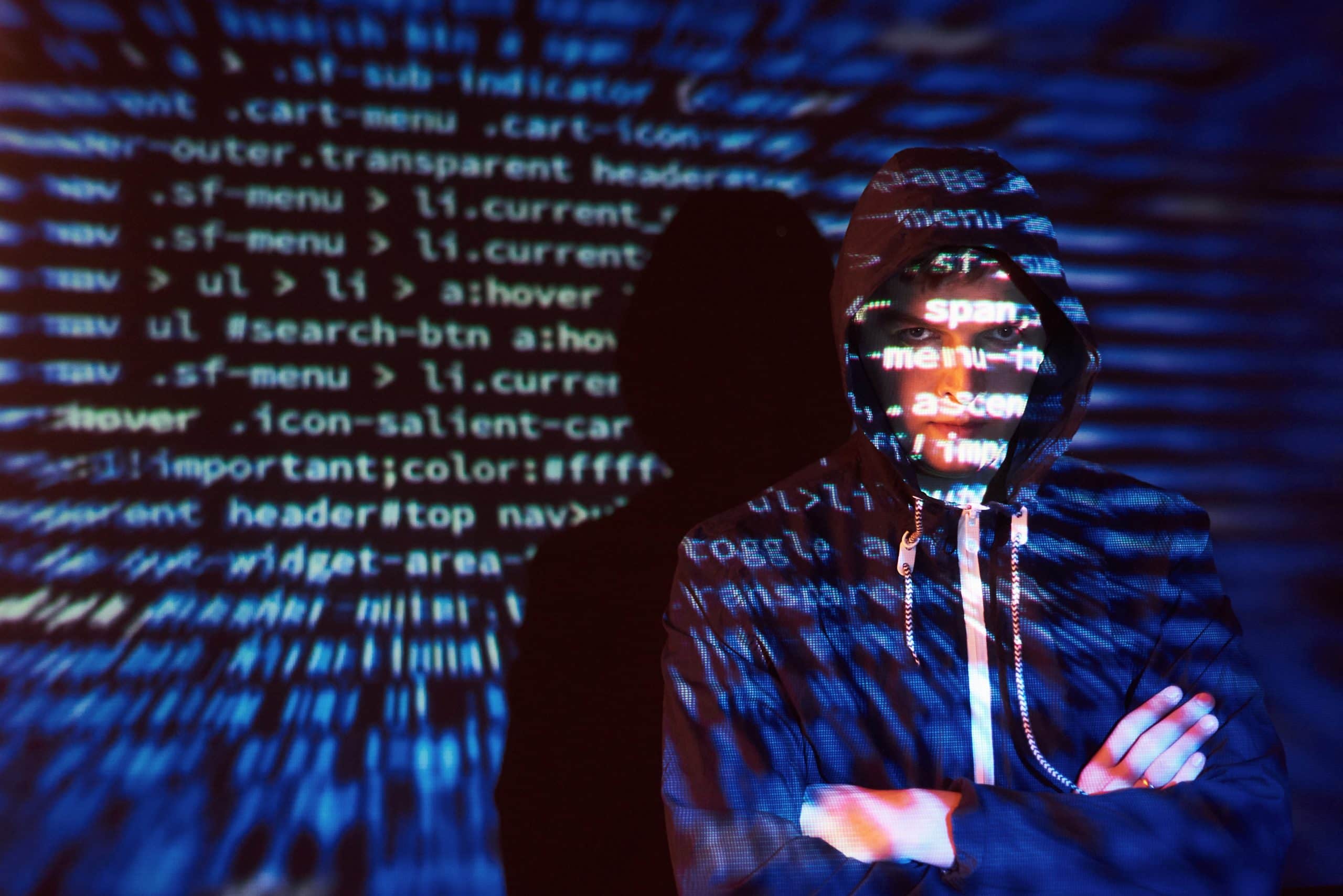
Data Privacy
Among the key issues that arise when applying Artificial Intelligence and Data Science together is data protection. Dealing with confidential data entails applying strong measures to minimize the risk of unauthorized access. These measures help prevent exposure to the data. Businesses are bound by data protection laws such as GDPR and CCPA. They must also switch to better security measures to protect personal and sensitive information.
Bias and Fairness
AI makes decisions, and if such decisions are unfair, it only amplifies the existing unequal disparity between different groups of people. This can impact numerous real-life decisions. To tackle the problem of bias, it is necessary to employ multiple and unbiased datasets and incorporate fairness algorithms. Additionally, frequently auditing the AI models and systems is crucial for maintaining fairness.
Integration Complexity
The capability of implementing AI in current and future Data Science processes has its problems at the technical and operational levels. It is therefore imperative to have the right strategy and approach when integrating Artificial Intelligence technologies with data infrastructure. Some of the challenges that organizations need to deal with include issues of compatibility and data quality.
Skill Requirements
The integration of AI and Data Science makes it necessary to hire a workforce that comprehensively understands the two. It may well be possible to meet difficulties in recruiting competent professionals who have the kind of knowledge required in machine learning, data analysis and data engineering. To promote AI and Data Science transformation within an organization, it is crucial to develop a skilled and competent team that can drive the change.
Future Trends and Developments
Evolving Technologies
This field of Artificial Intelligence and Data Science is still young and the progress that is going to be made is huge. Such technologies as advanced neural networks, quantum computing, and generative AI are expected to improve the capacities and stimulate progress. These technologies have the potential to increase data processing rates and accuracy. They also enhance problem-solving capabilities, leading to new and improved uses and opportunities.
Collaborative Approaches
It is expected that future advancements will exhibit proper integration of artificial intelligence and data scientists in various business industries. More innovative solutions will come from cross-disciplinary teams of machine learning, data engineering, and domain experts. This will be an effective model in which new solutions to global problems as well as fresh opportunities will be found with the help of joint efforts and systemic thinking.
Ethical and Regulatory Considerations
Ethical and legal challenges will grow as technologies like AI and Data Science evolve. The emphasis will be on the policy level to come up with ethical standards and regulations on the use of AI technologies. To ensure public trust and adherence to the rules, organizations will have to address changing requirements for transparency, accountability, and the use of data in the future.
Conclusion
The synergy between Artificial Intelligence and Data Science is recasting industries through upgrading the ways of information processing, prediction, and automation. However, this integration creates the potential for innovation and enhanced efficiency. It also introduces challenges related to data protection, bias in techniques, and system compatibility. In the future, the integration of AI and Data Science is expected to deepen with advancements in technology. By adopting these changes and managing the associated challenges, organizations will be able to capture the potential of this exciting synergy. This approach will help them fully leverage the benefits of integrating Artificial Intelligence and Data Science.