In the blooming era of machine learning, data is the primary entente to develop intelligent systems. However, the process of data collection, categorization, and storage is certainly not an easy and inexpensive task. In comes Snorkel AI which is a groundbreaking platform that is changing the way data is dealt with in machine learning. The future of machine learning is painted by Snorkel AI as a field that can embrace data labeling automation and faster model creation.
The Challenge of Data Labeling in Machine Learning
Before diving into how Snorkel AI is changing the landscape, it’s important to understand the critical challenge it addresses: data labeling. Like other machine learning processes, it requires a set of labeled data for training the model in the traditional classification settings. However, manual labeling of data is a time-consuming process which is also highly reliant on human intelligence and resources. When dealing with massive datasets, it becomes a time-consuming process and hinders the creation of machine learning models and raising the cost.
Independent entities label the data used to test machine learning models; thus, the quality of labelling becomes crucial. This results in long crawling and uncomfortable voyages, while incorrect labeling implies the necessity for further rounds of labeling and re-training. This cycle is not only time-consuming but also costly, which hinders organizations from scaling their AI projects.
Snorkel AI: A New Approach to Data Labeling
Snorkel AI meets these challenges by embracing a different approach to data labeling using weak supervision and programmatic labeling. Instead of the costly and time-consuming process of manually labeling data, Snorkel AI lets data scientists define labeling functions—short programs that apply rules, heuristics, or patterns to label data automatically. Of these labeling functions, those can be done fairly quickly and can be applied to large datasets and thus is much cheaper than doing it manually.
It is also important to note that weak supervision used by the platform refers to the use of numerous low-quality, partially, or imprecise sets of labeling information to develop high-quality labeling datasets. By aggregating these sources and modeling their errors, Snorkel AI provides labels which are as good as if not better than labels produced by traditional manual methods. It not only speeds up the labeling process but also enhances the quality and uniformity of the labeled data.
Accelerating Model Development and Iteration
It is also evident that Snorkel AI offers one of the most substantial benefits of speeding up the model-building and refining process. In previous processes, data scientists need to independently categorize a significant amount of information before the training of a model could even start. This first phase can sometimes last for weeks or even months, primarily due to the size of the dataset and the difficulty of the labeling process.
When using Snorkel AI, data scientists can obtain labeled datasets in a matter of hours, allowing for faster model prototyping. This kind of accelerated development cycle makes it possible for the organization to try out various models and strategies concurrently and make decisions faster. The flexibility in labeling functions and the capability to easily switch between labeling enables the improvement of the models which ultimately yields better performance and results.
Democratizing Access to Machine Learning
The second revolutionary event is Snorkel AI’s capability to bring machine learning to the masses. While creating and implementing machine learning schemes in the past, it was crucial to have professional skills in data science and the particular field. This volume of manual data labeling made it seem like only big companies or those with large budgets could invest in AI.
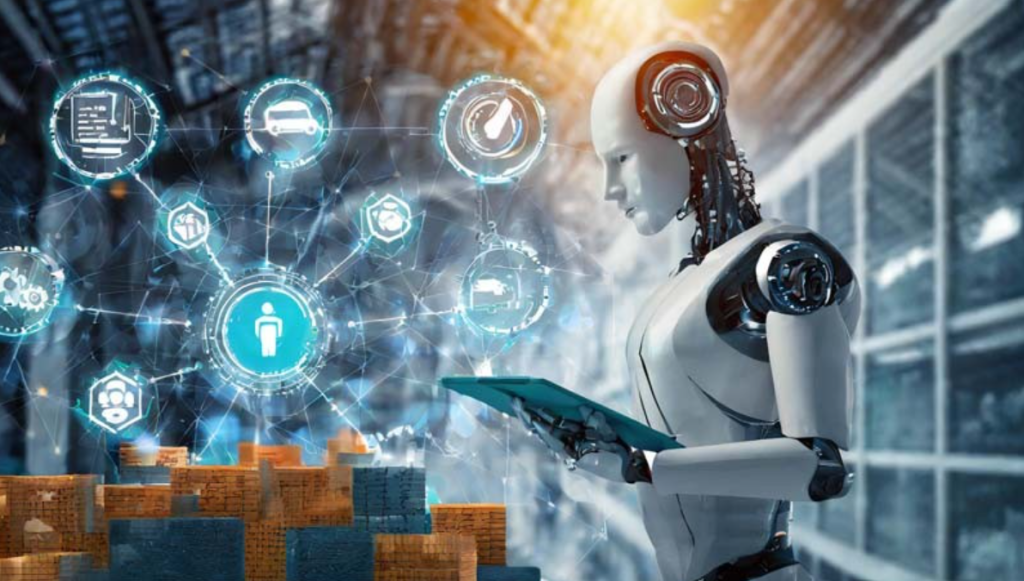
Snorkel AI addresses this issue because the tool breaks down the data labeling process and makes it less complex for people who do not have deep knowledge of it. Due to the ability to use the programmatic labeling technique, it is very simple to label programs regardless of the level of labeling skills. It makes it accessible to small organizations, startup businesses, and even individuals thus promoting the growth of machine learning and AI technology
Enhancing Model Transparency and Interpretability
Not only that, Snorkel AI also improves model understanding and explainability, which are fundamental issues affecting the uptake and deployment of AI solutions. In typical machine learning workflows, the labeling process is opaque, and there is little insight into how labels are produced or why some of them are generated.
Snorkel AI’s programmatic labeling concept eliminates the opacity that accompanies most traditional labeling procedures. As labeling functions and rules are set, it becomes easier to monitor the labeling practice with regard to the planned objectives and ethical guidelines. This sort of transparency makes sense in fields where regulation is strict, as with model decisions in healthcare or finance.
Furthermore, Snorkel AI has a weak supervision setting that enables the integration of domain knowledge and expert advice into the process of labeling. With the help of labeling functions, organizations can effectively incorporate specifications of domain knowledge into the model. This results in more accurate and realistic models that are closer in nature to real-world applications.
The Future of Machine Learning with Snorkel AI
However, high-quality labeled data will remain highly valuable as machine learning advances in the future. As a leader in the data labeling space, Snorkel AI leaves no doubt that the company will continue to play an essential role in the development of machine learning in the future.
In the future, Snorkel AI will deliver improvements in natural language processing, computer vision, and other Artificial Intelligence subdomains. Some of the uses of the platform involve building basic to advanced AI models and improving existing models or systems.